2020-12-18: Squad Call: Retrospective, Summary of What We Accomplished in 2021, and What We're Doing Next
Recording: https://iu.mediaspace.kaltura.com/media/1_8xg6i7yd
Summary: Thank you, everyone, for making some of this squad's objectives a success. We have made quite a significant milestone and hope to see great results come 2021. For those who could not join our last 2020 meeting, take a look at the retrospective and roadmap. Feel free to add to the list or upvote any item. Also here is the slide containing a summary of the retro and the accomplishments so far: https://docs.google.com/presentation/d/1f0VFO0eucWZYpIDOtm8smcCNWdW_7YjdhBWS7bdfTJ8/edit?usp=sharing
2020-12-10: Squad Call
Recording: https://iu.mediaspace.kaltura.com/media/1_4sqok6f1
Updates
- Allan -
- Piotr - setting up test sync via docker w/ HAPI FHIR server example to stream to. Reverting back to R4; only using R3 for buntzen part of pipeline. Currently doesn't incorporate a number of the fixes he made to the atomfeed client.
- Ian - generating some test date (goal is to finish January); Allan to follow up with statistics on variables
- Cliff - end-to-end test for changes (moving debezium settings to an adjacent file), PLIR: working on an e2e test for the changes made to enable the hapi fhir JPA support basic authentication
- Moses - connecting to OpenHIM with FHIR2 module work; presenting today
Topics
- ReadMe file too bulky
- Demos
- Need to continue Spark API discussion
2020-11-24: Standup
Recording: https://iu.mediaspace.kaltura.com/media/1_oj89pvy1
2020-11-19: Squad Call
Attendees: Bashir, Burke, Jen, Allan, Ayesh, Daniel, Ian, JJ, Jorge, Kenneth, Joseph, Sharif, Steven, Suruchi
Recording: https://iu.mediaspace.kaltura.com/media/1_ksxewulx
2020-11-17: Standup
Recording: https://iu.mediaspace.kaltura.com/media/1_yb0q1jsq
2020-11-12: Spark & Parquet prototype demo, and how to query them to generate indicator data
Attendees: Bashir, Ayesh, Brandon, Cliff, Daniel, Ian, Jacinta, Jen, JJ, Kenneth, Allan, Grace, Joseph, Mozzy, Piotr, Sharif, Steven, Vlad Shioshvili
Recording: https://iu.mediaspace.kaltura.com/media/1_dpyhepf4
- Dev Updates
- Bashir - PRs and prototype of indicator calcs plus spark implementation
- Cliff - PRs - moving debezium to DB config; adding javadocs to specs we have in AE code; getting rid of some runners for streamin. PRs wiating waiting for merge:
- Ian - trialing generating stats from Bashir's prototype - script we can plug weights into and it's a matter of getting the %'s right (in R)
- GitHub repo for weighted scripts coming
- Allan - to share more comprehensive statistics; had to make some changes recently
- Piotr - End to End testing the PR for HAPI FHIR communication with Sync: batch and atom feed streaming approach; ongoing issues w/ end to end FHIR tests; set up w/ Docker but atomfeed not installing on it due to liquibase problems. Transporting resources with a summary tag to HAPI FHIR server → HAPI FHIR server was not allowing resources with a summary tag to be saved; had to remove the tag to send.
- Jacinta
- Working on the end to end test for the pipeline
- Todo
- Switch from using OpenMrs nightly image to latest which is stable
- Piotr requesting addition of atom feed module
- Re-generade Demo Data
- Steps to generate demo data:
- The steps for generating demo data are documented here: Demo Data. It’s basically a matter of setting a single global property and restarting an OpenMRS instance. And then waiting
- Prototype Demo: Implementation of Indicator Calculation
- Why Parquet: Benefits of using Parquet
- Columnar format: Using parquet files because of columnar format - can go through those columns without actually fetching those records. Huge difference to other systems. Parquet is an open source version based on Dremnel which was originally an internal Google tool.
- Considers distributed environment: When you have huge amt of data you can't store on a single machine. You shard file into many shards, that can be distributed across many different machines.
- If you have 10's of workers - they can filter out the data they have, and just fetch the records that are of interest for our query. So you don't see benefits when running locally, but you do when it's distributed.
- Compression:
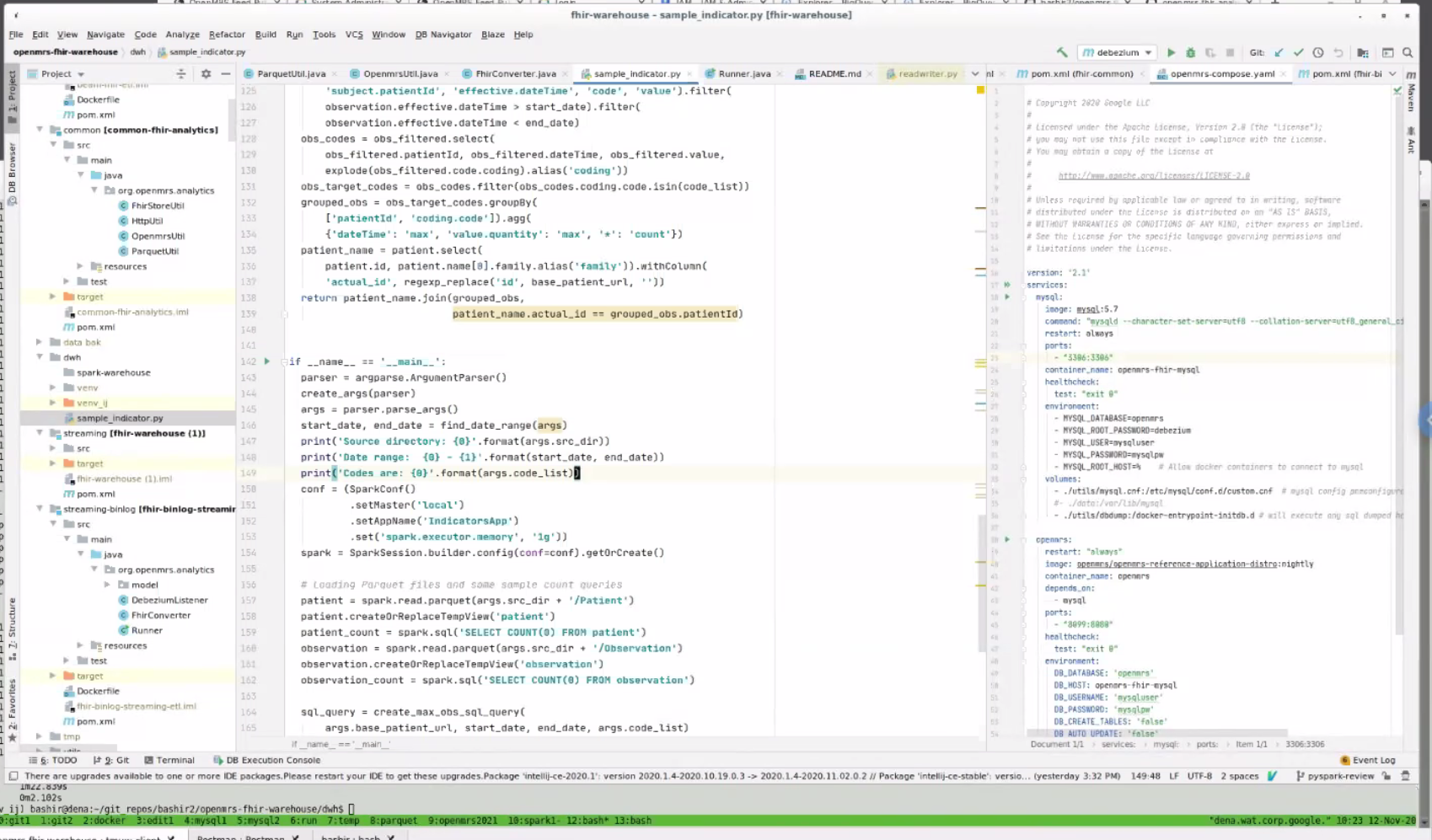
- Apache Arrow: Apache uses this internally to represent parquet files
- Why Spark
- Map-Reduce efficiency: typicaly map, shuffle, reduce- most of the intermediate steps involve writing to disk. With this approach, things go 10-100x faster than a usual map-reduce.
- PySpark = Python API to spark; so this demo is mostly Python. Can also create Jupyter notebook that talks to PySpark (Bashir just isn't doing it that way yet).
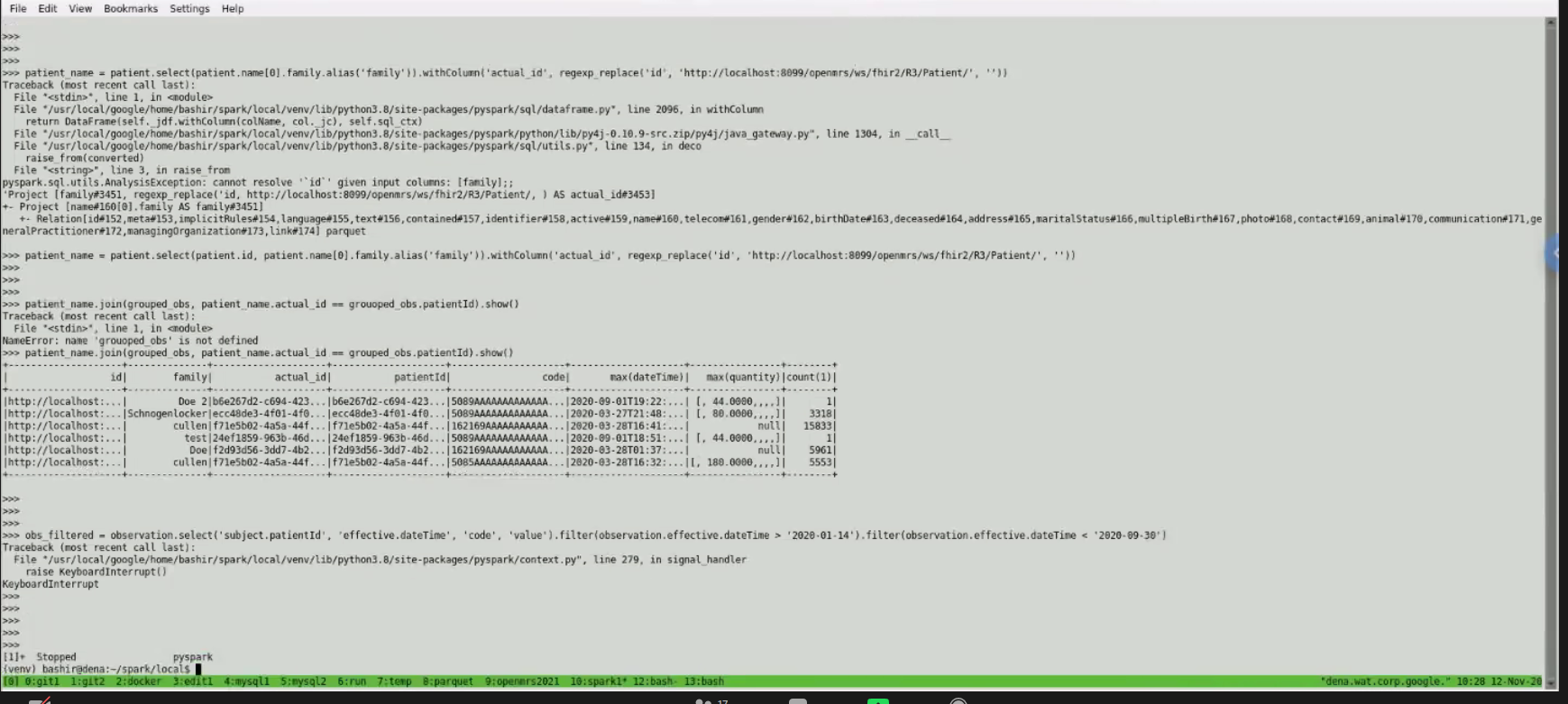
- Think Locally and still be able to scale:
You submit your query to spark. Beauty = the same thing (the spark - submit sample indicator) can be sent to a distributed cluster - means you can think locally, but continue to run when you scale to a distributed setup. Can use this exact same command to run on local or on real Spark cluster. - Example: Looking for Codes

- Option #1: Write query with SQL code and FHIR:
- Can structure indicators with FHIR codes. Someone who knows FHIR and knows SQL - it should hopefully be easy for them to write these queries

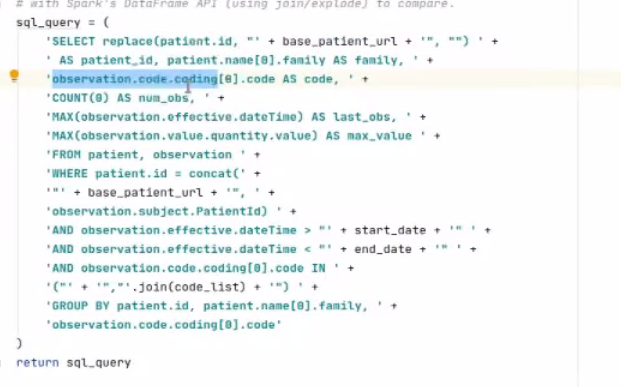
- Option #2: via Python querying Spark API
- Using Python to query Spark dataframe which is seamlessly distributed on multiple machines
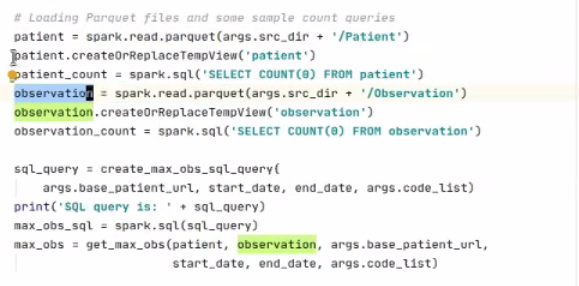
- Can just focus on columns of interest:
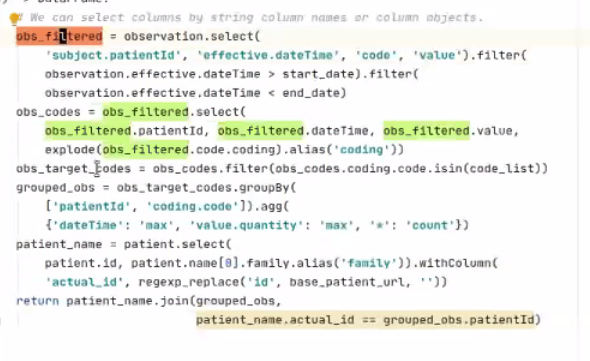
- Pros/Cons of the 2 Approaches
- Performance: SQL query may have done better; unclear b/c small test set size
- Learning Curve: Spark API took 2 days to learn how to query their API.
- Readability: with SQL, clearer to see if you're calculating things correctly. SQL is more likely to be readable/familiar.
- Maintenance: Spark API query more maintainable than SQL query as your queries grow. E.g. creating an API into our own server to query indicators could save time longer term.
- TODOs
- More feedback next week
- Slack discussion on Option 1 vs 2, and Python vs Java
- Create Google Cloud infrastructure a some kind of notebook to query it
2020-11-10: Standup
Recording: https://iu.mediaspace.kaltura.com/media/1_km9wjf5z
2020-11-05: Squad call
https://iu.mediaspace.kaltura.com/media/1_xwiwud01
2020-11-03: Standup
Recording: https://iu.mediaspace.kaltura.com/media/1_52jeny9e
2020-10-27: Standup
https://iu.mediaspace.kaltura.com/media/1_jm4887sf
2020-10-22:
Recording: https://iu.mediaspace.kaltura.com/media/1_0f2p04pj
Attendees: Allan, Bashir, Burke, Grace, JJ, Suruchi, Daniel, Ian, Jacinta, Jorge Quiepo
Welcome to Suruchi, sr dev from NepalEHR with interest in implementing analytics engine at their implementation, and new OpenMRS PM/Dev Fellow.
Reminder: Squad Showcase next week
Dev updates:
- Bashir has been working on simplficiation of camel
- Allan
- Converting atom feed config service logic
- Performance assessment of batch (patient, obs, encounters)
- Needs to add additional FHIR resources
- Bashir command line flag - can specify resources + search (this is because batch mode built on top of search api)
- OpenMRS Docker file has problems - liquidbase migration is happening despite update_db setting at false
- Jacinta
- Moses
2020-10-15:
Attendees: Bashir, Grace, Cliff, Daniel, Ian, Jacinta, Juliet, Allan, Mozzy, Piotr, Sharif, Vlad, Jen, JJ
Recording: https://iu.mediaspace.kaltura.com/media/1_h7p70dss
Dev. updates:
- Piotr - finishing up my couple of PR by next week, on the streaming docker setup and HAPI fhir client use
Addition of 2 new devs to team
- Moses Mutesa - fellowship mentor
- Cliff - volunteer
MVP Progress
- Want to have a way to transform data from an OpenMRS intance, in batch and streaming modes, trans data into FHIR that's easily converted into Spark, so that we can run queries at scale.
- Success metric: When we can run FHIR queries on spark at scale.
- Batch issues have been resolved and can create in Parquet.
- In a few weeks, how much to focus on original 10 PEPFAR metrics, given current CQL discussions? Can expose Metrics API that can be consumed. More updates to come from Vlad in next 2-4 weeks. If we integrate some of their work, means we'd be able to map to ~15 indicators.
What's the next step for getting from FHIR to indicators?
- (1) Next 2-4 weeks, focus remains on getting FHIR queries to run in Spark at scale. Getting engine running (as opposed to just a few metrics) - once that's running, (2) try implementing the example list of metrics, (3) look at whether there's an automated way of handling metrics like those examples in the future.
- Squad needs to focus on (1), the mission-critical groundwork.
- Summary: Datasource: OpenMRS. Tool to get it over: Parquet. Structure: FHIR. ETL Query tool: Spark. Means analyst can set up workspace to run ad hoc queries quickly at scale that run in seconds without impacting production. (E.g. every patient that visited my clinic within the last few months with HIV) Future: To be able to use other analysis/warehousing tools, more work to do.
Ampath example: SQL scripts generate report values. E.g. How many patients are on ARV: Take QID 123 and QID 345.
So - is it fair to assume that almost everything else but the concepts is the same? This is why Vlad's mapping tool is of great interest. Users need to be able to back up through the logic to show the result can be trusted.
How do we represent those SQL case statements? Can add logic into your FHIR module. E.g. my unified viral load concept
Ian investigated how TX_PVLS was calculated in 3 different implementations. All 3 were using slightly different definitions, even when using the exact same concepts. Some stored as obs, some stored as orders.
How PLIR/Notice D work is Different
- Indicators calculated in OpenHIM; focus is on passing FHIR resources through
- Prove extraction of the relevant dataset in the right format
Who's doing what next?
- Demo for implementers at next Squad Showcase
- Examples of a few calculations - Ian?
- JJ to share SQL case statements with Vlad & Squad
2020-10-08: Presentation of Analytics Engine approach and work so far & FHIR background
Recording: https://iu.mediaspace.kaltura.com/media/1_4ievjc2p
Attendees: Allan, Bashir, Burke, Ashtosh Padhy, Cliff, Daniel, Kenneth, Michael Gehron, Vlad Shioshivili, Ivan N, Piotr, Suruchi, Tendo, Ian, Juliet, Moses, Sharif
Overview presentation of the Analytics Engine Squad's work so far, & discussion with Michael & Vlad from PEPFAR PLM team.
PPT: https://docs.google.com/presentation/d/1ZCa3VXOtOvNB442YqB91kS5zkMTjDdjn7AI1ApwaP-g/edit#slide=id.g8f74bf3fe7_0_48
- Emphasis from squad on performant solution that can handle large quantities of data - this drives our architecture decisions. Planning to access info through a Metrics API.
- FHIR was chosen as the API for talking to OMRS. FHIR is also the main data model for the Analytics Engine to benefit more from standard tools built on top of FHIR.
- FHIR2 module/bundle can generate FHIR resources, so Analytics Engine can rely on that; default information model is FHIR.
- The fhir2 module is currently a series of translators that transform into FHIR. Long term hope is to replace stuff we're storing in custom OMRS formats with FHIR, so we speak FHIR natively. Goal of module for now is to transform 80% of that custom OMRS data into FHIR.
- The FHIR2 module update recently released: Reading the vast majority of data in OMRS - can read patients, orders, conditions; some writable parts of API still WIP.
PEPFAR logic changes happen so frequently, so the PLM work is aiming to include a logic model that they (PEPFAR?) would keep up to date so implementers could just reference that, and not have to each go through the massive work of logic updates.
2020-09-24
Present: Vlad Shioshvili, ALlan, Bashir, Christina, Daniel, Burke, Ian, Ken O, Michael Gehron, Tracy, Grace, JJ, Jen
Recording: (PEPFAR PLM demo starts 14 minutes in) https://iu.mediaspace.kaltura.com/media/Analytics+Engine+Squad/1_4759yb4v
Agenda:
- Discussion on CQL and PEPFAR metrics.
- Update: Progress with FHIR schema and Spark - not done; next time
- Update on overall Squad progress: the analytics squad has created prototypes for extracting data using FHIR in both batch and streaming modes. However, we have not done any work on indicator transformations/aggregation
- Decision: Decide btwn Debezium embedded and Camel Debezium - plan was to use Debezium directly, but Allan has done some tests and Camel seems like a better idea - so this PR is going forward.
- PR for adding basic auth (username/pwd) from Piotr.
@Vladimer Shioshvili continued the presentation on PEPFAR's Patient-Level Monitoring for M&E Proof of Concept as well as gave a live demo. Slides: https://docs.google.com/presentation/d/1t9astN7NboSycFax0vNgZxVJ2Tc6OJF3_3HJaJGGAIM/edit
- Questionnaire resource mapped to FHIR - collects questionnaire responses which are also mapped to FHIR.
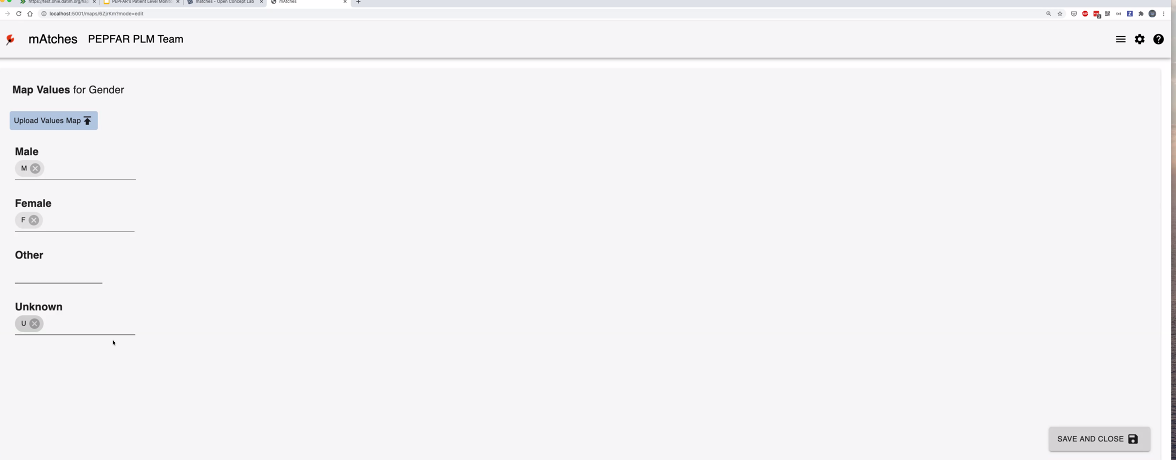
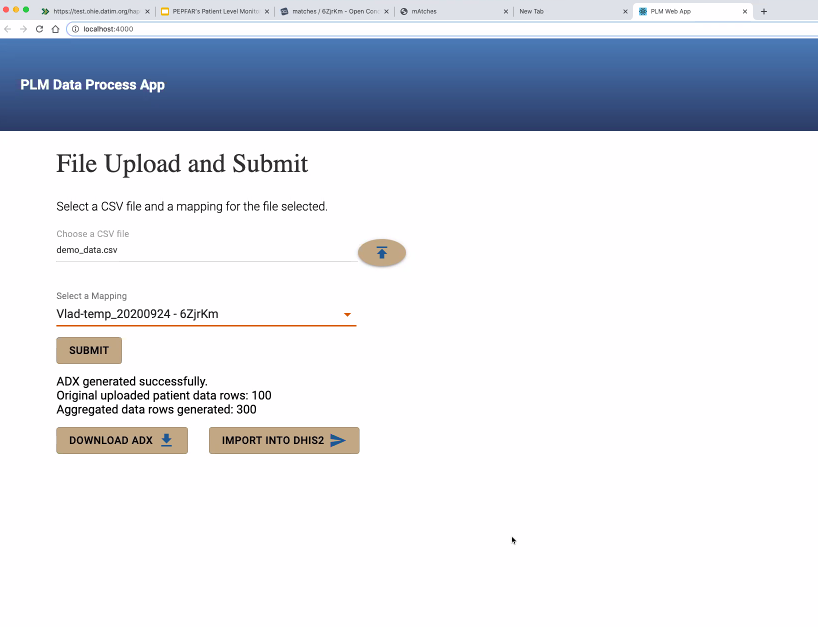
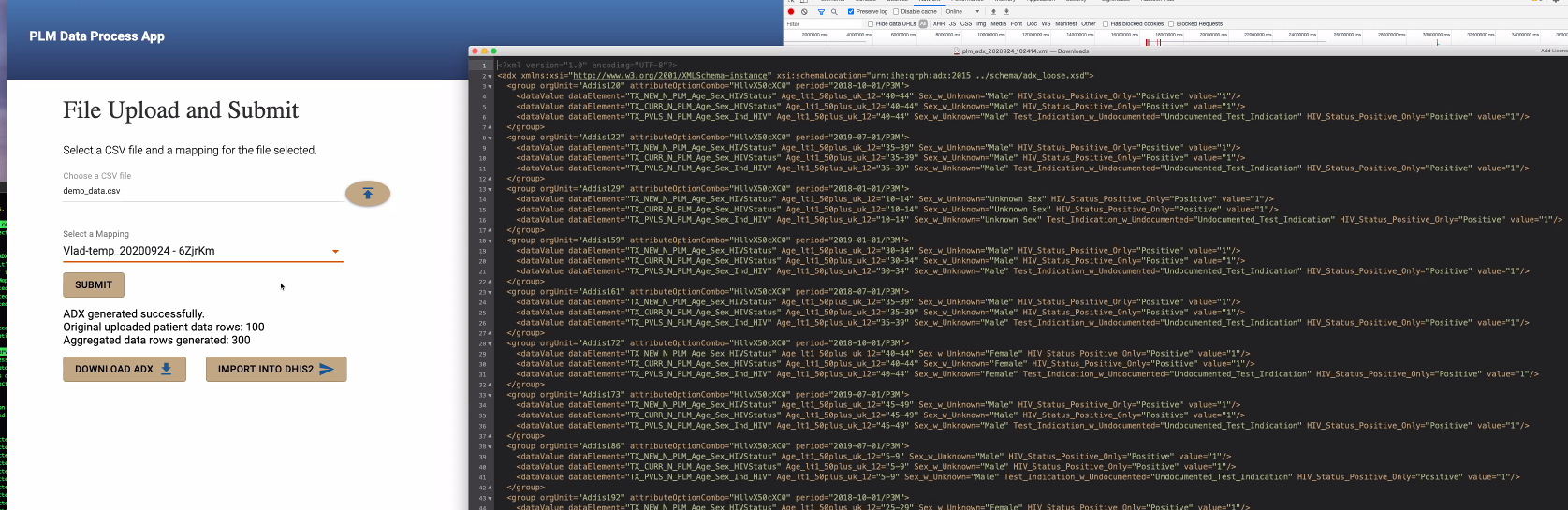

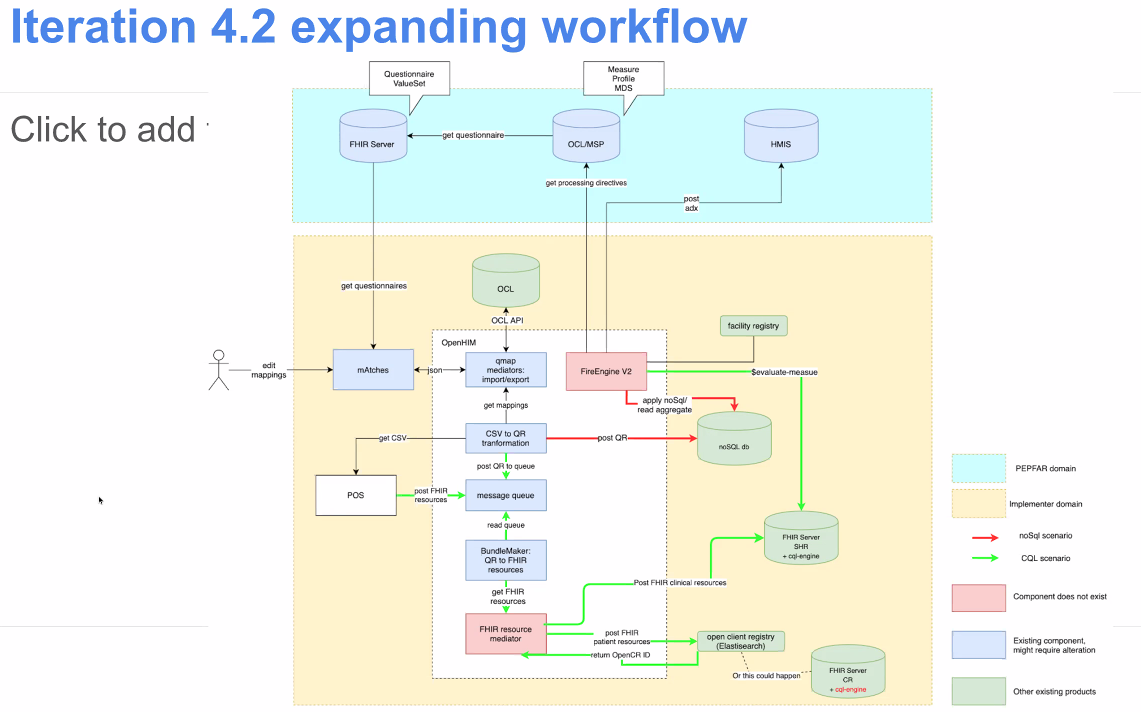
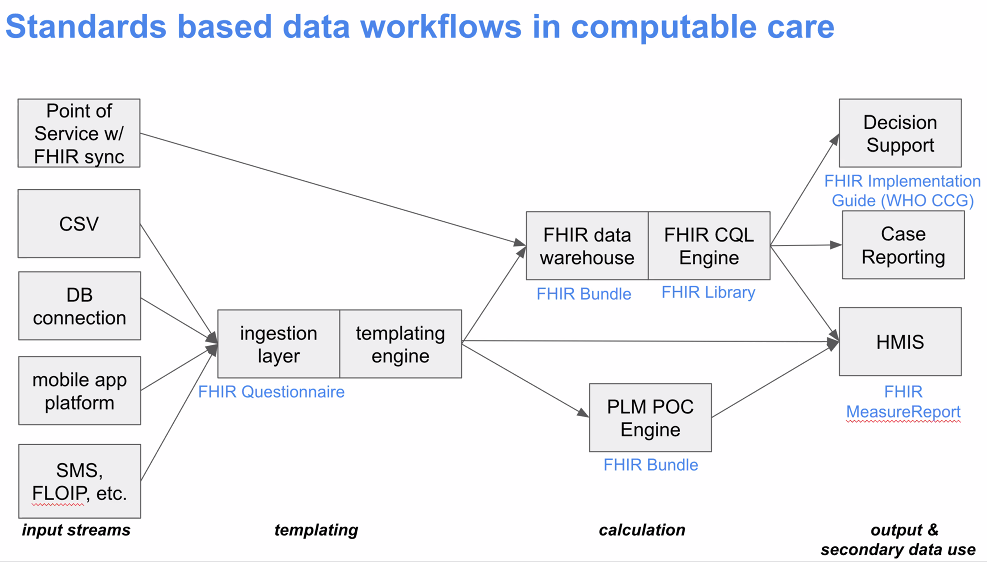
Working with IntraHealth on integrating the openclient registry to reconcile the patients across different point of service applications. So when they receive messages from multiple systems, want to make sure one person is indeed counted as one person. A lot of times, false positive LTFUs happen because of a transfer of a patient to another system, that didn't get captured as one patient moving.
Looking to use CQL instead of custom agreggations they have written right now.
Should be able to share Repos within a couple of weeks.
Next Steps:
2020-09-17
Agenda:
- Demo from group from OpenHIE's Data Use Community (DUC) who want to generate MER indicators on FHIR
- Update: Progress with FHIR schema and Spark [next time]
- Decision: Decide btwn Debezium embedded and Camel Debezium [next time]
2020-09-10
Present: Allan, Bashir, Burke, Daniel, Debbie Munson, Ian, Jen, JJ, Juliet, Mozzy, Sharif, Tracy, Grace
Recording: https://iu.mediaspace.kaltura.com/media/1_gkdr9uvr
- Announcements
- Product Management update - JJ Dick will begin co-leading Product Management of the squad with Grace
- Call timing update - please do this poll: https://doodle.com/poll/hy8ekhwxy9n3m7ck so we can make this call accessible for more people
- Other announcements or work people would like to update the squad about?
- Allan: to demo his progress
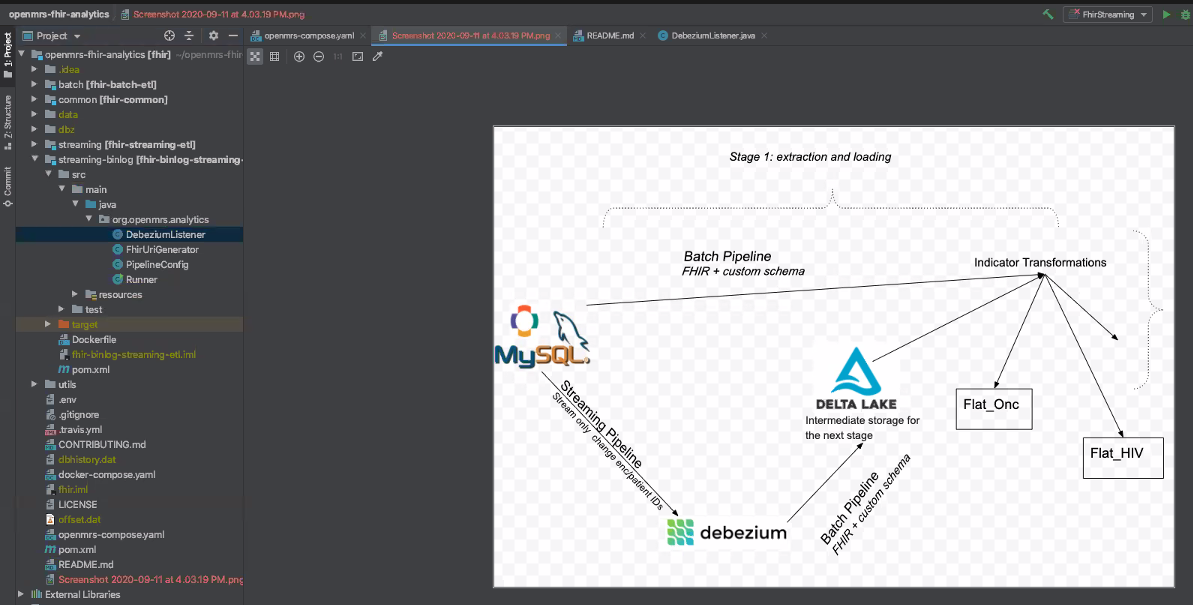
- Takes < 3 mins to extract 3,000 datapoints from ~500 encounters; tested with encounter and obs
- Asks a lot of the FHIR2 module for performance (current focus of FHIR squad has been resource mapping, not performance, yet); could be further optimized
- Allan suggestion: People should be able to extract the data however they want, but FHIR as the preferred/recommended mode
- Next steps: How can we move forward and get work done together?
- Schema Talk Thread: https://talk.openmrs.org/t/to-schema-or-not-to-schema-deciding-on-a-foundation-for-our-analytics-engine/29953/11
- Problem: Need to have flexible engine, for where FHIR won't help us (e.g. where a new table cannot be mapped to FHIR, or you have a separate labs DB which you want in your analytics warehouse where you can query it). For Ampathy, FHIR will work ~80% of the time, but may not work all the time. So: Do we want to go for schema or schema-less warehouse?
- Allan's recommendation: Support both - FHIR as main schema, and have flexibiltiy to store data, have your analytics engine access that
- Bashir: For first prototype, use SQL on FHIR. You can still bring any type of data into the warehouse that's not FHIR. Current prototype uses FHIR API to pull the data. Eventually we could bring an extension into the pipelines that creates a view, brings the data forward anyway. Once you have custom data in your warehouse that you want to use for analytics, then by definition, you have to use custom logic anyway.
- Burke; FHIR not ubiquitous enough, don't want to lock ourselves in.
- Mapping to FHIR is not a requirement.
- Decisions
- Agreement: We're moving forward with at least FHIR.
- What's next? Try moving forward with Spark.
- Debezium? Discuss next time.
- New meeting time: Thursdays at 2pm UTC
- TODO: JJ to intro squad to other group from OpenHIE's Data Use Community (DUC) who want to generate MER indicators on FHIR; try to get them to demo next time
2020-09-04
Recording: https://iu.mediaspace.kaltura.com/media/Analytics+Engine+Squad/1_iw9pijl5
- Kenneth Ochieng (Intellisoft) shared work for DS Notice D as proof of concept system for integrated approach to patient-level reporting
- Using Bahmni for point of care system
- Using Bahmni's Atom Feed Module to expose data
- Sending patient data using FHIR format to OpenHIM
- Storing FHIR data into HAPI FHIR server within HIE as shared health record (SHR)
- Using FHIR Measure resources within HAPI FHIR server
- Will use CQL engine against HAPI FHIR server to calculate measures and share them with DHIS2
- Defining development process
- Making decisions: use Decision Making Playbook
- Slack channel: #analytics-engine
- Handling tickets
- Consider using github during prototype stage
- May re-visit and consider JIRA once a shared approach & repo(s) has been decided
- Repository
- Handling Pull Requests
- Would like to have a server within OpenMRS for testing (e.g., ae.openmrs.org for "analytics engine")
- Large demo dataset is ~200 GB
- At least 8 GB (16 GB would be preferable)
- Could repurpose sync servers
- Make the call about data store and general structure/approach.
2020-08-28
Recording: https://iu.mediaspace.kaltura.com/media/1_zo38ckft
- Discussion on the road-map; request for feedback
- How to process data?
- We have a bias toward Apache Spark
- Tools for handling data workflow
- Delta.io is a tool allan kimaina has been evaluating
- Apache Beam was suggested by Bashir Sadjad
- Bunsen is an interesting tool backed by Cerner that allows
- Would like to aim for initial examples
- Using FHIR Data for a PEPFAR report using FHIR
- Use a custom datatype in early examples to demonstrate that the pipeline is not FHIR-only
- CQL support
- CDC seems to have a preference for PowerBI - we can expect this to be a priority use case (exposing or transforming data into a format that PowerBI can use)
- Stretch goal: Running PythonNotebook on top of data
Action: Make the call before or by next call about data store and general structure/approach. allan kimaina & Burke Mamlin owning follow-up.
2020-08-21
Recording: https://iu.mediaspace.kaltura.com/media/1_rxkt7fss
Attendees: allan kimaina , Bashir Sadjad , Burke Mamlin , Ian Bacher , Jennifer Antilla , Juliet Wamalwa , Kenneth Ochieng, Piotr Mankowski , Tracy, Grace Potma
- Dev. updates
- Batch implementation pushed to same GH repo. Demo'd recently to FHIR squad. https://github.com/GoogleCloudPlatform/openmrs-fhir-analytics
- Piotr working on dockerized version of streaming app to synchronized between an OMRS and HAPI FHIR instance
- POC needed in 6 weeks - so Piotr working on getting atomfeed solution cleaner/simpler
- Review of MVP goals
- OMOP/schema feedback
- Proposals for issue tracking, code location, and code review (TL;DR; GitHub)
- Agreed on using GitHub for issue tracking in this squad
- Bashir on Vacation next 2 weeks
2020-08-14
Attendees: Antony, Burke, Allan, Bashir, Christina, Daniel, Debbie Munson, Jayasanka, Jen, Juliet, Tracy
Recording: https://iu.mediaspace.kaltura.com/media/Analytics+Engine+Squad/1_7yw4tayq
- Welcome!
- Antony (Palladium), Debbie (PIH)
- Announcements
- Dev. updates
- Bashir: Code for monitoring changes, transforming data later into a FHIR resource. Opensourced now
https://github.com/GoogleCloudPlatform/openmrs-fhir-analytics- Location: Google Cloud Platform for now while still getting started. Apache License. Can go to OMRS if that's a better place in future.
- Please check it out!
- Allan: Evaluating w/ Cornelius (Ampath FHIR expert) - went through data tables. Found 70% of Ampath data represented w/ FHIR; what about other 30%? Looking to expand that.
- To use FHIR as intermediate storage, we definitely need a custom schema that covers that other 30%.
- Challenge: How to limit this so people don't depend too much on custom schema. This wouldn't realize the benefit of the entire pipeline.
- Some implementers not comfortable with FHIR - this would enable them to use custom schema. But, adds scope: Requires us to support two schemas.
- Continue discussion on data warehouse schema:
- Still need to discuss schema. E.g. OMOP.
- API to access schema? At Google, history seemed to be SQL was trusted, i.e., SQL as an API for accessing data. The context is BigTable to SQL based APIs migration.
- At the end of the day, the query complexities are still there, even if we pick a non-SQL API.
- For end-users: Agreed that consumers need to have tooling at the end that allows them to get the data out - even without knowledge of SQL.
- For under the hood - Schema Flexibility: Concern around committing to relational database approach if we don't have to
- Nice because people can bring whatever they want
- Hard because it will be harder to build things on top
- E.g. Palladium: does development, other players consume data. Need frameworks that empower those users to come up with their own reports. So many funding agencies that all have their own reports (e.g. CDC, USAID, DOD), have different report requests at least every week! Need to be readily consumable for ad-hoc reporting. Where everyone understands queries for what's in the system.
- Being able to perform ad-hoc reports could be an argument for SQL
- Not clear that OMOP has wider adoption than FHIR. Well adopted in clinical research community.
- Keep researching/asking? Or just start with FHIR?
- Using FHIR for data analytics tools is already happening. Unclear: Are people widely comfortable with OMOP? Easier to work with. Primarily designed for de-identified data.
- We always have the option for FHIR→OMOP in future as there are open source tools for that transformation.
- OMRS quite involved helping set up OHDSI community
- FHIR background is to support both data structure for SHR and for analytics
- Where do we go from here?
- TODO: Allan to review OMOP
- TODO: Grace to set up meeting w/ Squad & James/Carl re. ADX/OMOP advice
- TODO: Burke to start Talk conversation around initial schema (w/ link to Analytics on FHIR)
- Concrete MVP goals:
- Single machine pipeline, while easily scalable (note on MySQL bottleneck)
- Horizontally scalable warehouse
- Metrics API with a minimum set of indicators
- Implement the 10 common indicators; show people how quickly they can be calculated. Idea is this could be a separate module that uses the warehouse.
- Deploy as docker image; they get an end point they can query to issue queries to underlying data. But has to be scaleable. OMRS itself not horizontally scalable. MySQL would be the bottleneck for scalability in the data pipelines.
2020-08-07
- Recording: https://iu.mediaspace.kaltura.com/media/1_pzweel3o
- Attendees
- Updates
- TODO - Grace set up call w/ James K & squad tech leads
- TODO - Grace consolidate visuals of ETL demos from implementers
- Deidentification of randomized data set ongoing by Allan at AMPATH
- Bashir experimenting with converting data to FHIR resources and pushing to a FHIR warehouse; includes bulk-update. To make open source code available to all next week.
- This code can be used to extract OMRS data as FHIR resources. Generic tool, not just for analytics, even though original use case was to push to FHIR warehouses.
- Review of hot-spots for run times
- Proof of Concept
- Looking for 1+ more site
- Need to i.d. what the intermediate schema is that they're okay with (e.g. some for/against FHIR) - is there any other analytics schema out there that makes it easy to come up with the concepts around it?
- OK to use SQL as main interface? (commitment that end product would be used)
- Need to i.d. intermediate schema, assuming it's not FHIR.
- Just build and get feedback from prototype?
- Do we need separate mechanisms for standardized indicators?
- Need to get to a data warehouse where we can get to those specific features; maybe phase 2 we get to specific querying
- TODO: Tracy to post and s
- Other standardized schema examples: OMOP, I2D2
- OMOP use case suggestion: Different way to transform the data into a standardized format, to have common data for analytics. Another way of trying to do data analytics / warehousing at scale. Advantages is that because it's been standardized as a model, a lot of tools being built against it.
- Building on REST API FHIR store loses some of the advantages of storing in SQL
- Need to support not-cloud-based: We can provide tools (e.g. Spark), but responsibility of implementers to manage their own cluster if they want scalability beyond single node.
- Would be nice to provide these sites w/ different versions of Docker component files for such cases.
- "Engine"
- Deidentification
2020-07-31: First Squad Call
- Recording: https://iu.mediaspace.kaltura.com/media/Analytics+Squad/1_id8660id
- Attendees
- Burke Mamlin , allan kimaina , Bashir Sadjad , Christina White , Daniel Kayiwa , Dimitri R , Ian Bacher , @Jayasanka, Jen, JJ, Mike, Steven, Tracy
- Introductions
- Technical Leads Allan & Bashir
- Mekom: Keen to include analytic platform in work for clients
- Jayasanka: Working on DHIS2 reporting module, love for analytics/viz
- AMPATH: Have been supporting hacky ETL solution; excited to have more robust solution
- PIH: Often less than ideal approaches to ETL & analytics, excited for something that helps guide things forward
- KenyaEMR: Steve - historically has seen reporting solutions have all been suboptimal – e.g. having a ministry report running all night
- ITECH: Christina: Hoping to implement in Haiti. Tracy: secondary use of data at large scale; researcher of predictive analytics & clinical decision support
- Our prototype goals
- Clarify purpose as prototyping (it’s unknown what final tool/product will look like)
- Specific indicators
- Scalability (run on 1+ nodes)
- Goal is to create something useable soon (i.e., get to a working prototype people can actually use)
- Lessons learned from OMRS19:
- Common need for flattening encounters (e.g. people trying to analyze How many patients did we see where X happened?)
- Creating a table for every form - an idea from prototype approach that suggests how it will work to automatically produce e.g. a CSV for each form
- Lessons learned from BIRT & others:
- Our data model is constantly changing; the questions you want to answer are always new (nature of medical info - which test is done changes year to year)
- Need tooling that's at production level to not have to define our whole schema up front. Use standards & tooling conventions wherever possible.
- Need to echo standards so we don't have to build multiple aspects - tools coming to existence built on standards (e.g. expect to see more of this w/ FHIR). Want tooling we can support in 1-2 years.
- FHIR as (1) first intermediary step/data store w/ initial transforms, +/- (2) build your own extension as needed w/ transforms you need
- There will no doubt be efforts outside of OpenMRS to transform FHIR to OHDSI's common data model
- ITECH: working on prototyping way to pipeline data into a side-by-side HAPI FHIR store for q. OMRS instance - hope is to use some kind of ETL
- Bashir has been experimenting with extracting into FHIR schema
- Will need to discuss schema more!
- Leveraging recent community EIP work
- Debezium chosen as way to broadcast elements, create an outbound from OMRS to get data from
- Now need tool to help route messages (and well dev'd/designed, saves ++ dev time) - Apache Camel well-suited, but can't drop Camel on EIP side
- OMRS should be able to spit out FHIR messages out of the box. Until it can, need to identify the layer that does this and who's getting it done. Ideally FHIR subscription model is what OMRS should be able to do. So "if you need X data, subscribe to OMRS and FHIR model can generate for you". Mekom willing to support full-time developing ability for OMRS to support doing this.
- ETL tech layer should expect to receive FHIR data - not the job of the ETL pipeline. Extract FHIR and transform as needed from there.
- Need reliable event generating system. That's what the pipeline pulls from. (Right now in OMRS this is basically MySQL)
- How often we meet
- Weekly
- First priorities:
- Requirements & what's getting built - ground in prototypes groups have already made
- One prototype every squad call? (20% of time on prototypes every week)
- Send doodle (or Slack poll) for ?Wednesday, next presenters
- Sprint structure
- Where we collaborate digitally
- We use Talk for discussions and decision-making (documenting decisions)
- We use Slack #etl to chat (and assume those discussions will never be seen again)
- Data environment
- Centralized server with unified sample dataset that we can use to do development and analysis
- Cloud vs On Premise
- Any other role clarification
2020-07-30: Focused discussion on PIH's ETL work
Attendees: allan kimaina Bashir Sadjad Ian Bacher Grace Potma Mike Seaton Mark Goodrich
- PIH's ETL infrastructure:
- https://github.com/PIH/petl
- Chose Pentaho because concern about need for other devs who understand more cutting edge tech if they chose a different option.
- This tool executes pipelines; Pentaho separates them. Analysts using PowerBI to create reports; don't write in SQL. PowerBI couldn't directly suck data from MySQL. Approach was crashing machines of end users; and it became clear that PowerBI was going to be what end users were using to review analytics. PIH added a MySQL to MS SQL-server bridge since PowerBI can consume data from SQL-server directly.
- Is the goal to calculate some fairly standard metrics or do they vary from site to site? They vary and there data analysts who are not SWEs but know how to use data, e.g., through SQL. They may have custom needs.
- Another source of variability is minor differences between different OpenMRS implementations.
- FHIR:
- Requiring FHIR would be a big concern because not useable/friendly enough for end-user Analysts.
- No way the users of their analytics tools would be able to write a query like this:
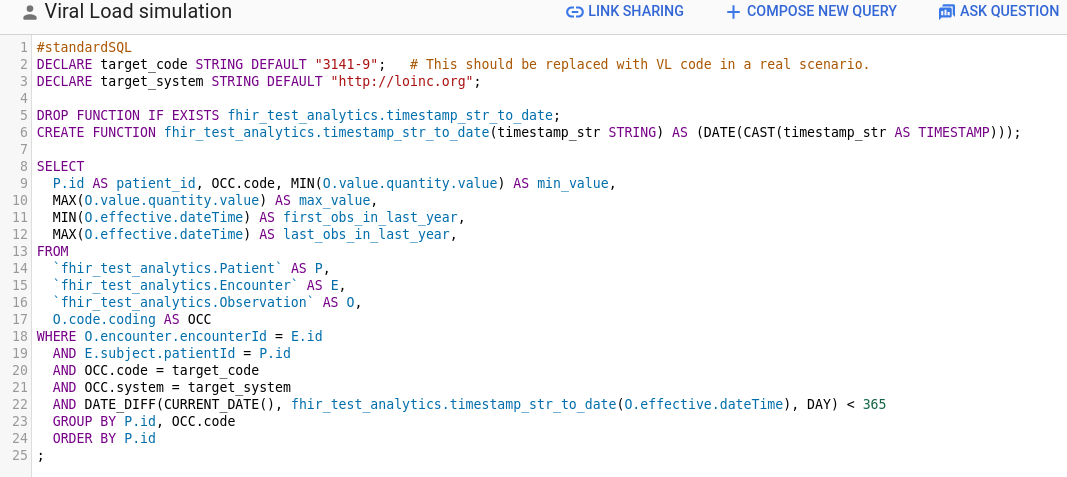
- But we need some middle schema anyways, can that middle schema be FHIR? Potentially, yes. It also depends on the adoption of things like SQL-on-FHIR.
- There is an agreement that if we want the middle layer to be flexible enough to do various analysis, its data model and queries will be inherently complex.
- The current main pain points:
- How to get data out of OMRS; currently it is through cron jobs that recreate data warehouse tables from scratch. Incremental support is definitely useful.
- Dealing with OMRS data model is not easy.
- Is the current PowerBI system work on a single machine or a cluster? How many patients and observations are we talking about?
- Yes, PowerBI runs on a single machine.
- One of PIH's bigger installations has ~400K patients and ~40M observations.
- People want to be able to drill down to actual data, so just providing the target metric is not enough.
2020-07-16: Identify considerations for squad success
Attendees: allan kimaina Bashir Sadjad Ian Bacher Jennifer Antilla Grace Potma
- Need for a separate non-MySQL platform?
- Might be fine when you have small, single sites; but when you want to do data analysis on all them, you need a data warehouse where a single MySQL approach won’t work
- Bashir developing things beyond MySQL already - but do we really need a non-MySQL-based solution?
- Work of squad could be just to write those scripts
- Aim: Not to be locked-in to MySQL. GSoC project working on support for Postgres b/c right now other groups having to install MySQL just to run on OMRS
- Unclear: How possible is it to have a solution that runs across different SQL servers? (SQL supposed to be a standard but reality is it gets implemented differently across multiple vendors → problem for OMRS)
- Posed these questions in Bashir’s FHIR Analytics Google Doc
- Calculations of concepts in background end up causing headaches - this ETL approach doesn’t scale @ Ampath; KenyaEMR have similar experience
- Takeaway: Not going to be a single MySQL approach, because this doesn’t scale and doesn't address the issues IN’s have already run into
- Final output and its usage
- People used to writing SQL - need a tech that someone in M&E/Data Science can simply write SQL to generate a report
- Requirement: Whatever we do, ppl need to be able to run SQL on the solution
- Spark? Hive? Others from Hadoop+?
- Small deployments (10’s of clinics) - should be easy for them to use in single processing environment (on device on location); shouldn’t assume everyone knows how to bring up a cluster w/ 10’s of nodes
- Output could be a MySQL DB
- Requirement: Whatever metric is required, there should be support to drill down further, all the way down to 1 record (easily able to go down to pt records)
- E.g. “Aggregate the # of pts with VL Suppression.” Ppl see # and say “this is an under/over report!”
- Ability to return to MySQL
- Requirement: It should be easy to use the data from within OMRS (exposing the data in OMRS should be easy)
- Quickest way is through MySQL. But exporting data back into MySQL can become a bottleneck
- Module could be the reporting module, tweaked to be able to work with this.
- Requirement: Data in warehouse should be de-identified
- Feature? Issue is compliance.
- Alternative: Access Management
- Deployment requirements
- MVP Requirement: Runs on single node
- MVP+ Requirement: Runs on multiple nodes
- Everything should just work regardless of how many VMs (single or 10’s) - does not require/assume cluster management. E.g. shouldn’t expose dataflow clusters to end users
- MVP Requirement: Provide simple notebook with examples on how to use, query, specific transformations
- Don’t assume ppl know how to run spark cluster or connect data to mySQL DB
- Others working on ETL: UW+I-TECH, Mekom, PIH, etc.
- I-TECH: Meeting w/ Jan & Tracy (to work on I-TECH’s ETL work)
- Mekom: DB Sync work - separate binary that syncs multiple OMRS deployments
- PIH: Mike & Deb & Mark re. How they’re using COVID19 as case study for ETL pipeline
- These are the ppl to regularly engage with when we set up squad (even if all they do is attend weekly squad meetings to give feedback & contribute)
- Team/work/processes etc.
- Clarify purpose as prototyping (it’s unknown what final tool/product will look like)
- E.g. weekly vs q2 weeks, with open collaboration session other week
- Sprint structure
- We use Talk to ____
- We use Slack to ____
- Centralized server with unified sample dataset that we can use to do development and analysis
- Cloud vs On Premise
- Requirements of external agencies - e.g. @ Google all OS forked repos must live under a Google Org (would hurt visibility)
- Bashir to f/u at Google.
- Intro of Technical Leads
- Our prototype goals
- How often we meet
- Where we collaborate digitally
- Data environment
- Any other role clarification
- Work tracking process (Bugs, tickets) while working towards prototype?
- How we consolidate our code, code review process & how we expect this to change over time; expectations for merge/release frequency
- Address nuances contributors are working with
- Decision: Best if everything we develop lives under OMRS.
- Time offering
- Rules of thumb we’ll follow for decision making
- For reference: Decision Making Playbook
- Who has decision making authority
- What to do when lack of consensus is blocking forward movement
- Champion sites (these would be the people who'd use our outputs when we release them)
- Main champion site at this point = Ampath b/c they have biggest DB (& Allan’s expertise!) - able to say “with a DB this size, we can run these obs and metrics successfully” so it should work for smaller sites
- Elevator pitch: Solution that shortens the time and improves the quality of using OMRS data in indicator reporting, reduces unplanned technical team member overtime, and makes it easy to drill down to patient-level data to confirm the numbers are correct.
DONE: Grace post announcement on Talk w/ Doodle; Grace combine requirements into Requirements page.